Materials Informatics
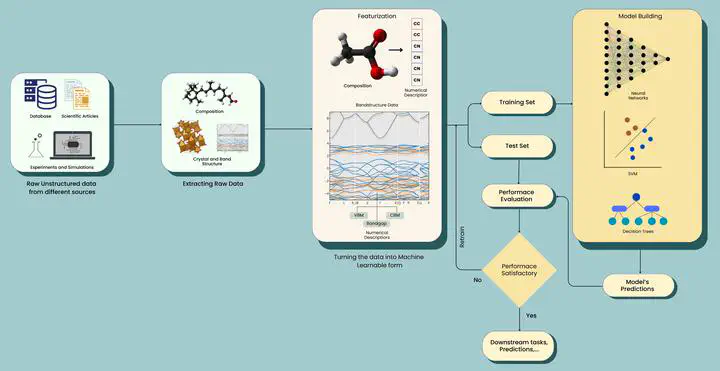
The discovery of novel functional materials underpins the modern technological revolution, yet identifying materials with specific properties within an immense chemical composition space remains a formidable challenge. The complex structure-property relationships in materials make this search inherently difficult. Traditional approaches, such as trial-and-error experimentation or high-throughput ab initio methods, while reliable, are time-consuming and resource-intensive. These limitations hinder rapid exploration across the vast design space, slowing down the pace of discovery.
At MAVENs, we address this challenge by leveraging Machine Learning (ML) to revolutionise materials discovery. ML introduces a data-driven paradigm, enabling the identification of intricate patterns within chemical and structural datasets. By learning from training data, ML models make rapid, physics-informed predictions about material properties, significantly streamlining the discovery process. Unlike traditional approaches, ML offers the potential to explore vast chemical spaces efficiently, reducing computational costs and accelerating innovation. To overcome the challenge of “black-box” models, which sacrifice interpretability for performance, we develop hybrid ML approaches. These methods integrate predictive power with physical interpretability, mapping feature vectors to physical descriptors. This transparency ensures our models provide both accurate predictions and meaningful scientific insights.
One example of our success lies in designing ML models for materials tailored for quantum information processing. We focus on predicting defects in materials analogous to the nitrogen-vacancy (NV) centre in diamond—defect systems with electronic levels suitable for physical qubits. These materials have the potential to address key challenges in building arrays of qubits with long coherence times at room temperature. Our models achieve remarkable accuracy, with an F1 score exceeding 0.98 for classification tasks and a Matthews correlation coefficient above 0.90 on imbalanced datasets, all while preserving interpretability